Statistics Data Analysis: A Thorough Examination
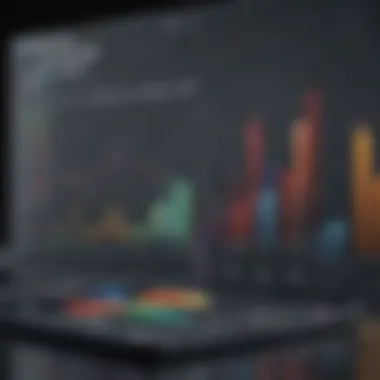
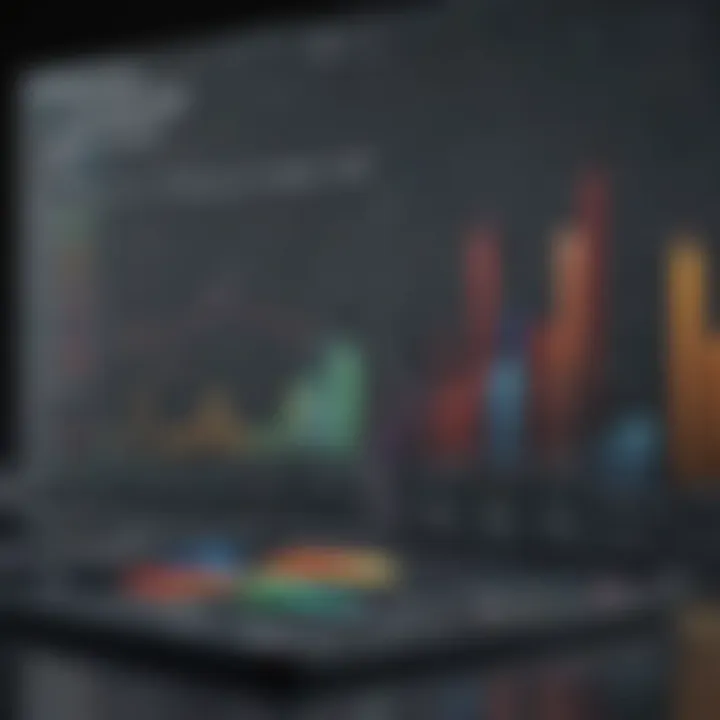
Intro
Statistics and data analysis form the backbone of informed decision-making in a world overflowing with information. As the quantity of data grows at a dizzying pace, understanding how to interpret that data has become not just advantageous, but essential. Whether in business, healthcare, or even social sciences, the ability to extract meaning from numbers opens doors to new insights and innovative solutions.
This article aims to illuminate the core aspects of statistics data analysis, catering to both the rookie who’s just stepping foot into the world of numbers and the seasoned analyst who wants a deeper dive into methodologies and applications. We’ll cover the essential tools, discuss how to visualize data effectively, and identify common pitfalls along the way.
Moreover, engaging with ethical considerations in data analysis is crucial as we navigate this complex landscape. As we look to the future, understanding evolving trends will keep us ahead of the curve, allowing us to leverage data for actionable insights and sound decisions.
With that in mind, let’s start by getting familiar with some standard terminology that frequently arises in the realm of stats and data analysis.
Investment Terminology
Statistics data analysis can be opaque to the uninitiated. Let’s clear the fog by defining key terms that are essential in grasping the broader concept of data analysis.
Definitions of Common Terms
- Descriptive Statistics: These are methods for summarizing and describing the main features of a dataset. Measures like mean, median, and mode fall under this category.
- Inferential Statistics: This approach involves taking a random sample from a population to infer trends or predictions about the whole population. It's where statistical tests and confidence intervals come into play.
- Data Cleaning: The process of correcting or removing inaccurate records from a dataset. Think of it as tidying up; you can’t make decisions based on sloppy data.
- Data Visualization: The graphical representation of information and data. Visualization tools make it easier to understand complex data sets and reveal patterns that are less obvious in traditional numeric forms.
Industry Acronyms and Abbreviations
In any industry, acronyms and abbreviations proliferate. Below are some common ones you'll encounter in the field of statistics and data analysis:
- SQL: Structured Query Language, a standard programming language for managing and manipulating databases.
- EDA: Exploratory Data Analysis, an approach to analyzing data sets to summarize their main characteristics often using visual methods.
- ANOVA: Analysis of Variance, a method used to compare means among three or more groups to see if at least one is significantly different.
"If you can’t measure it, you can’t improve it." – Peter Drucker. This truism is particularly relevant in statistics, where measurement is at the heart of data analysis.
By arming ourselves with the right terminology, we set the stage for a deeper exploration into the methodologies and tools that will anchor our understanding of statistics data analysis. As we transition into the next segment, we’ll dissect the strategic use of these methodologies and their real-world applications.
Defining Statistics
Understanding statistics in depth is pivotal when exploring data analysis. Statistics is not just a series of numbers or mathematical functions; it is the backbone that informs decision-making in diverse domains such as finance, healthcare, marketing, and social sciences.
Statistics ultimately transforms raw data into meaningful insights. It provides frameworks for gathering, interpreting, and presenting data. It's akin to turning a blank canvas into a striking piece of art, where each brushstroke represents data points, variables, and relationships.
Moreover, embracing statistics enriches our comprehension of data. By defining this field, we can appreciate its role in various applications, recognize its strengths and weaknesses, and equip ourselves with the tools necessary for effective analysis. It's not just about crunching numbers; it's about making sense of the chaotic world of data.
Overview of Statistics
Statistics is a discipline that follows a systematic approach to collecting, analyzing, interpreting, and presenting numerical data. To put it plainly, it's all about making sense of data, whether from surveys, experiments, or just observations of life. The power of statistics emerges when messy data is organized and analyzed.
Beneath this broad field, statistics can be divided into two primary categories: descriptive and inferential. Each serves distinct purposes but complements one another.
Types of Statistics
Descriptive Statistics
Descriptive statistics provides a compact summary of data through various metrics. Think of it as a scalpel that slices through data to reveal essential insights while discarding the fluff. The key features include measures of central tendency like mean, median, and mode, as well as measures of variability such as range, variance, and standard deviation.
The major benefit of descriptive statistics is its ability to represent large datasets in a digestible format. For instance, a company might summarize its monthly sales data to understand trends over the year. This approach allows stakeholders to make informed decisions based on clear facts.
However, it’s not without limits. While it paints a helpful picture, descriptive statistics does not allow for conclusions beyond the data observed. It merely describes what is there, no more.
Inferential Statistics
Inferential statistics takes the concept further. It involves making predictions or inferences about a larger population based on a sample of data. This is crucial when it's impractical to collect data from every individual in a population. Techniques like hypothesis testing and confidence intervals are central here.
One standout feature of inferential statistics is its capacity to generalize findings from a sample to a whole group. For example, polling data before an election draws conclusions about voter behavior across an entire region, not just those surveyed. This ability makes inferential statistics invaluable in research and analysis.
However, the guessing game comes with its own risks. Interpretations drawn from inferential statistics can lead to false conclusions if the sample is biased or the assumptions underpinning the analysis are flawed. So, it’s essential to tread carefully.
"Statistics is the belief in the averaging of ideas."
In summation, defining statistics sets the stage for deeper insights into data analysis. Descriptive statistics helps simplify, while inferential statistics allows for broader generalizations. Together, they form a crucial foundation for interpreting data effectively, which will be explored throughout this exploration.
Understanding Data Analysis
Data analysis serves as the backbone for making informed decisions based on gathered information. Understanding its principles and processes can transform raw data into meaningful insights. It goes beyond mere number crunching; data analysis encapsulates defining problems, collecting and preparing data, interpreting results, and ultimately applying these findings in real-world contexts. This article aims to demystify this multifaceted topic, offering insights that cater to both novices and seasoned experts.
Prelims to Data Analysis
At its core, data analysis is the process of systematically applying statistical and logical techniques to describe, illustrate, and evaluate data. Imagine having a treasure trove of information at your disposal; without the proper tools and techniques to decipher it, that potential remains locked away. Data analysis acts as a key, unlocking possibilities that can drive strategy and inform key decisions.
To break down the process, consider the following steps involved in data analysis:
- Problem Definition: Before diving into the numbers, it's crucial to have a clear understanding of the questions you want to answer. This sets the stage for effective analysis.
- Data Collection: Gathering relevant data from appropriate sources ensures the analysis is grounded in reality. It can include surveys, experiments, and database mining.
- Data Cleaning: Raw data rarely comes to you free of imperfections. Cleaning data by removing duplicates, inconsistencies, or incomplete entries is vital to ensure accurate analysis.
- Data Interpretation: This step requires critical thinking. Here, statistical tools come into play to derive meaning from the cleaned data.
- Communication of Results: Presenting findings in a clear and compelling manner is essential. Visualization techniques often aid in illustrating complex insights in an accessible format.
The importance of understanding these steps cannot be overstated, whether for academic research, business decision-making, or personal investments. Data analysis fosters critical thinking and enhances one's ability to discern patterns and correlations that might otherwise go unnoticed.
Importance of Data Analysis
Data analysis carries immense weight in today's information-laden world. Its significance extends across multiple domains, including business, healthcare, and social sciences. Here are some key aspects illustrating its importance:
- Informed Decision-Making: With accurate analyses, organizations can make decisions based on facts rather than assumptions. This leads to better resource allocation and improved outcomes.
- Efficiency and Productivity: By identifying inefficiencies, organizations can streamline operations. Think of data analysis as a magnifying glass, highlighting weak spots that need attention.
- Risk Mitigation: Understanding trends and anomalies through data can help businesses identify potential risks before they become critical problems. This proactive approach saves time and money.
- Predictive Insights: Leveraging historical data patterns enables organizations to forecast future trends. This predictive capability is a game-changer in various industries, from finance to retail.
- Competitive Advantage: Companies leveraging data analytics tend to stay a step ahead of their competitors. In today’s fast-paced economy, timely access to information can be the difference between thriving and merely surviving.
In summary, understanding data analysis empowers individuals and organizations to harness the full potential of the data around them. Recognizing its value is just the beginning; the challenge lies in executing the analysis carefully and responsibly to draw insights that can drive progress.
"Better data leads to better insights. Better insights lead to better decisions."
As you delve deeper into this article, the exploration of different techniques and tools will further enlighten your understanding of the expansive world of data analysis.
Statistical Methods in Data Analysis
Statistical methods are the backbone of data analysis, providing a framework for making sense of the vast amounts of information at our disposal. These methods allow researchers, analysts, and decision-makers to draw conclusions, test hypotheses, and make predictions based on data. The significance of employing the right statistical techniques cannot be overstated, as they directly affect the reliability and validity of the outcomes. From businesses analyzing market trends to scientists exploring patterns in research data, statistical methods cater to a variety of needs that span numerous fields.
Common Statistical Techniques
Statistical techniques are the instruments used in this broady spectrum of data analysis. Each technique serves a unique purpose, and understanding their functions is crucial for getting the most out of any analysis.
Regression Analysis
Regression analysis stands out as a vital statistical approach for examining relationships between variables. In essence, it helps assess how one variable changes when another one does. This method is often used for prediction and forecasting. A crucial aspect of regression analysis is its ability to fit a mathematical model to the data, revealing valuable insights while keeping the noise at bay.
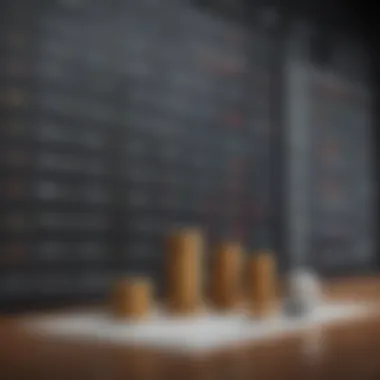
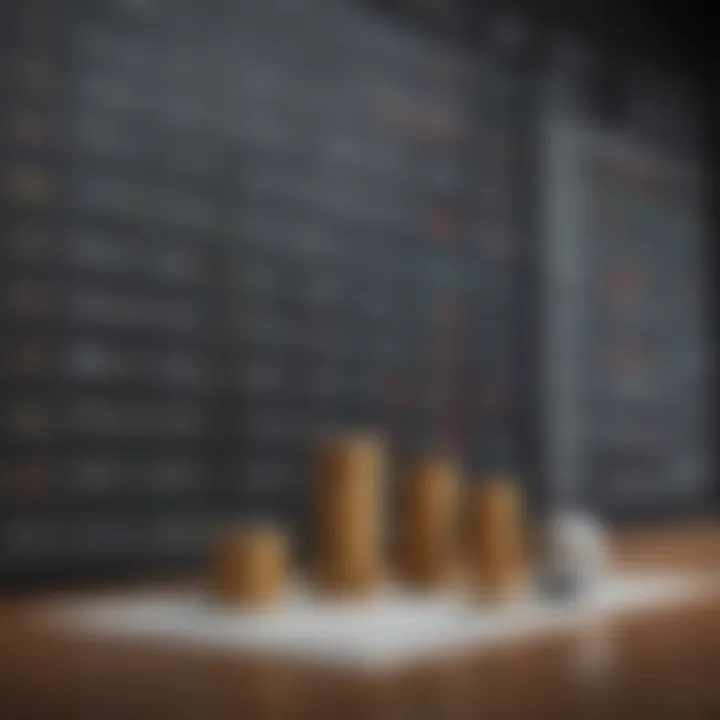
One of the key characteristics of regression analysis is its flexibility. You can apply it in multiple scenarios, from linear regression—which focuses on linear relationships—to more complex models like polynomial or logistic regression. This adaptability makes it a popular choice for analysts looking to explore associations in their datasets.
The unique feature of regression analysis is its ability to yield coefficients that quantify the relationship between the dependent and independent variables. While its advantages include intuitive interpretations and predictive power, the method can fall prey to overfitting if too many variables are included, thus losing its effectiveness.
ANOVA
ANOVA, or Analysis of Variance, is another statistical technique integral to understanding differences among group means. This technique allows researchers to test hypotheses and determine if there are statistically significant differences between the means of three or more independent (unrelated) groups. The prominent benefit of ANOVA is that it provides a method to compare multiple groups simultaneously without increasing the risk of Type I error that arises from conducting multiple t-tests.
A critical aspect of ANOVA is its robustness against violations of some assumptions, making it a beneficial choice for data analysts who deal with real-world data, which may not always meet theoretical standards. In terms of unique features, ANOVA partitions the total variance into components associated with different factors. However, it does have its downsides, such as sensitivity to outliers and its inability to tell specifically which groups differ from one another without further tests.
Chi-Square Test
The Chi-Square test is a classic statistical method used mainly for categorical data. It assesses how likely it is that an observed distribution is due to chance. This method allows analysts to test the independence of two categorical variables, helping draw conclusions from survey data and experiments. Its key characteristic lies in its straightforwardness; you can compute it easily, even if you’re somewhat mathematically challenged.
A unique feature of the Chi-Square test is its non-parametric nature; no assumptions about the distribution of the data are necessary. This makes it a valuable tool when working with nominal data. However, limitations exist. For instance, the Chi-Square test requires large sample sizes for validity, which may not always be feasible in smaller studies. Moreover, counts should ideally be five or more in each category to maintain statistical rigor.
Choosing the Right Methodology
Navigating the world of statistical methods can feel like trying to find your way through a maze. Each technique has its own set of strengths and weaknesses, and selecting the right one depends on various factors. Key considerations include:
- Nature of the Data: Is it categorical or continuous? This influences the choice of method.
- Research Goals: Are you looking to determine relationships, group differences, or patterns?
- Sample Size: Some methods require larger datasets to deliver reliable results.
- Assumptions: Understand the underlying assumptions of each method to ensure that they fit your data properly.
The right statistical technique, when chosen carefully, can elevate the quality of data analysis, leading to fuller insights that are actionable in real life.
Data Collection Techniques
Data collection serves as the bedrock of statistics data analysis. It encompasses the methods and processes of gathering information to answer specific questions or to test hypotheses. Without accurate and reliable data, all subsequent analysis becomes moot, akin to building a house on sand.
Importance of Data Collection Techniques
The importance of choosing the right data collection techniques cannot be overstated. It can determine the success or failure of your analysis. A good approach ensures that the data gathered is pertinent, accurate, and representative of the population being studied. Moreover, poor data collection may lead to misleading insights, potentially impacting business decisions or scientific research.
Furthermore, various factors come into play when selecting data collection methods. Research objectives, the nature of the data, available resources, and time constraints all factor in. With the rise of technology, there's an array of techniques available today, but one must tread carefully—after all, not every tool is suitable for every job.
"Good decisions come from good data."
This often quoted phrase among data scientists emphasizes the critical relationship between data quality and decision-making.
Surveys and Questionnaires
Surveys and questionnaires are among the most widely utilized tools for data collection. They offer a structured way to gather data, often leading to statistical rigor and clarity in results. Designed correctly, they allow researchers to glean insights into behaviors, opinions, and preferences of a population.
Benefits:
- Cost-effective: Especially when conducted online, they can reach a vast audience without significant expense.
- Flexibility: They can be tailored to suit different demographics, making them versatile for various fields including healthcare, marketing, and social research.
- Accessibility of Data: Responses can often be collected and analyzed in real time.
However, crafting effective surveys requires skill and careful consideration. For instance, vague or leading questions can skew results. Furthermore, determining how to distribute the survey is crucial; relying solely on online platforms may alienate segments of the population who are less digitally connected.
Experimental and Observational Study Designs
Experimental and observational study designs are crucial for understanding cause-and-effect relationships. Each design has its unique strengths and weaknesses, impacting how results can be interpreted.
Experimental Designs:
In an experimental study, the researcher manipulates one variable to see the effect on another. This method is most common in fields like psychology or pharmaceuticals. Here are a few key features:
- Control: Researchers can control for confounding variables, enhancing the validity of the results.
- Randomization: Participants are often randomly assigned to either the experimental or control group, reducing bias.
Observational Designs:
In contrast, observational studies involve monitoring subjects in their natural environment without intervention. This design is typical in epidemiological research. Some significant points include:
- Real-world Insights: It provides perspectives on how variables operate in real-life settings, offering a broader context.
- Feasible for Certain Situations: Sometimes, it’s not ethical or practical to conduct an experiment, making observational methods the go-to choice.
In choosing between experimental and observational designs, researchers need to consider their research question's nature. It is essential that the chosen design aligns with the objectives to ensure that the results will be meaningful—after all, apples and oranges aren’t interchangeable when analyzing data.
Data Processing and Cleaning
Data processing and cleaning stand as a crucial aspect of statistics data analysis. It’s the foundation upon which reliable insights are built. Without the proper handling of data, the results of any analysis may as well be like a ship without a rudder—lost at sea, struggling to make sense of what it’s navigating through. This phase ensures that the data is not only accurate but also organized in a way that enables effective interpretation.
Data Cleaning Techniques
When it comes to data cleaning, several techniques can help scrub the data clean, so it shines bright like polished silver. Common practices include:
- Removing Duplicates: Data often contains duplicates that can skew results. By identifying and eliminating these repetitions, accuracy is significantly enhanced.
- Handling Missing Values: Missing data is a common hiccup in data analysis. Depending on the context, one might fill in gaps with the average of the dataset, or simply exclude entries that are incomplete. Each strategy carries its own implications that need careful consideration.
- Standardizing Formats: Different entries might be recorded in varying formats. Standardizing ensures that all the data aligns and becomes compatible for analysis.
- Filtering Outliers: Outliers can throw a wrench in the works, causing misinterpretations. Identifying these outliers and deciding whether to investigate further or exclude them is important in maintaining data integrity.
These techniques are streamlined processes that prevent the dirty data from leading one down a misinformed path.
Data Transformation Methods
Data transformation takes the cleaned data and prepares it for analysis, making it turn from a rough diamond into a sparkling gem. This stage can include a variety of approaches, such as:
- Normalization: This involves adjusting the values in the dataset to a common scale, which can be pivotal when comparing different variables. Think of it like adjusting the volume on a speaker so that every instrument can be heard clearly without drowning out the others.
- Aggregation: Sometimes, data can be too granular. Aggregation combines data points to provide a more digestible overview. For instance, rather than analyzing every single purchase made at a store, one might aggregate sales by month to see broader patterns.
- Encoding Categorical Variables: In instances where data includes categorical variables, converting them into a numerical format can facilitate analysis. Techniques like one-hot encoding make it easy for models to process this kind of data effectively.
Data Visualization Principles
Data visualization is a crucial backbone to effectively understanding and interpreting statistical data. In an increasingly complex data landscape, the way we choose to visually represent information holds significant weight. Good visualization not only enhances clarity but also reveals insights that numbers alone cannot convey. Whether it’s illustrating trends, comparisons, or relationships, visual components guide decision-makers in grasping large sets of information quickly.
Effective visualizations tell a story, drawing attention to significant findings while guiding the viewer's focus. Misinterpretation can easily arise without suitable visuals, potentially leading to errors in judgment or action. Thus, it’s essential to invest time in creating visual aids that are both aesthetically pleasing and informative.
Importance of Visualization
The importance of visualization cannot be overstated in today’s data-driven world. Here are several key points highlighting its role:
- Enhancement of Understanding: Visuals convert complex data points into digestible formats that can be easily understood. For instance, a well-designed graph can simplify comprehension of trends over time, contrasting insights drawn from raw data.
- Identifying Patterns and Trends: By providing a visual representation of data, analysts can swiftly identify patterns or anomalies that may not be apparent in spreadsheets. This plays a pivotal role in predictive analysis and decision making.
- Facilitating Communication: Effective visualizations can serve as a common ground for discussions among stakeholders. They encapsulate findings that can be readily shared and evaluated, increasing the likelihood of consensus on analyses or strategies.
"A picture is worth a thousand words" – this adage resonates profoundly in the realm of data analysis, showcasing how visual tools can encapsulate tremendous amounts of data succinctly.
Popular Visualization Tools
With a plethora of visualization tools available, choosing the right one is crucial. Here are two of the most prominent tools known for their effectiveness and applicability in data visualization:
Tableau
Tableau stands out for its intuitive interface and powerful capabilities. One specific aspect of Tableau is its ability to connect directly to various data sources, allowing for real-time data updates. This feature ensures that users always have access to the most current data for their visualizations, making it a valuable tool in dynamic environments.
One key characteristic of Tableau that sets it apart is its drag-and-drop functionality. Users, even without advanced programming skills, can create complex visualizations with ease. This accessibility has made Tableau a popular choice among diverse professional sectors.
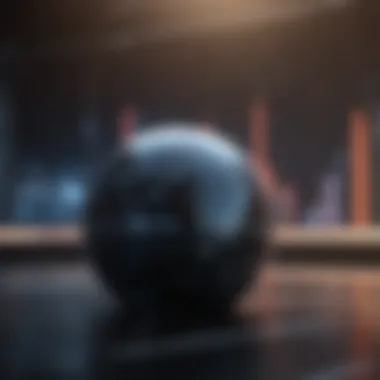
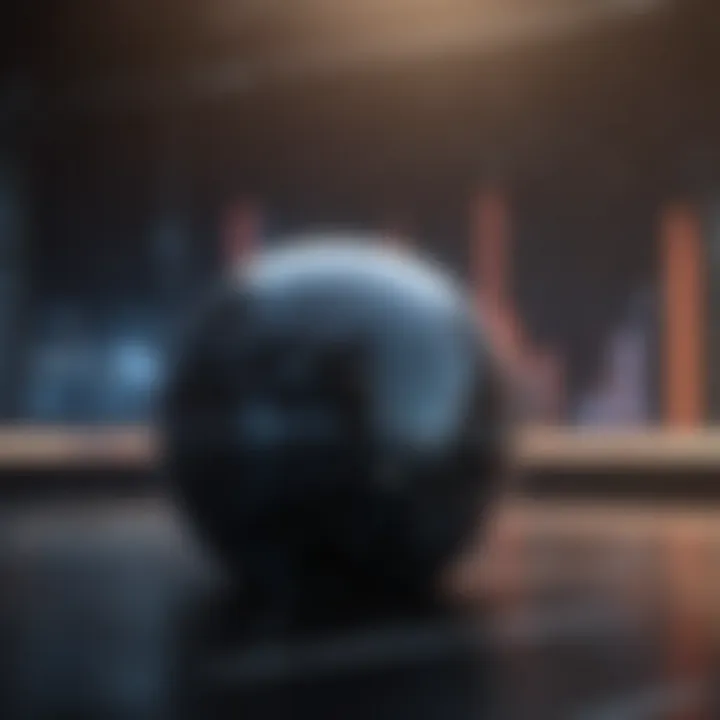
The unique feature of Tableau is its extensive library of visualization options, from traditional bar charts to advanced geo-mapping. The flexibility it offers regarding customization helps users tailor visuals to their specific needs, providing a distinct advantage in presentations and reports. However, a disadvantage might be the cost, which can be substantial for smaller organizations.
Power BI
Power BI shines in its ability to integrate seamlessly with other Microsoft products, which is especially beneficial for companies already utilizing Microsoft ecosystems. This integration allows users to pull data from various sources and create compelling reports effortlessly.
The key characteristic of Power BI is its user-friendly dashboard that allows for real-time data visualizations. Users can track important metrics at a glance, which aids in timely decision-making. Moreover, Power BI supports natural language querying, enabling users to ask questions about their data in plain language.
A unique feature of Power BI is its ability to embed reports into other applications, thus making insights widely available across an organization. However, compared to Tableau, it may not offer as much flexibility in design and the variety of visualization options.
By understanding the principles of data visualization and effectively utilizing tools like Tableau and Power BI, analysts can significantly enhance the impact and clarity of their statistical reports.
Interpreting Data Analysis Results
The ability to analyze and interpret data results is fundamental in making better-informed decisions. Whether in business, healthcare, or social sciences, understanding these results helps individuals and organizations pinpoint trends, relationships, and variances. It’s not just about crunching numbers; it’s about deriving meaning from those numbers that count.
This section digs into two pivotal concepts in data analysis: statistical significance and confidence intervals. These ideas lay the groundwork for effective decision-making, providing clarity on how to evaluate findings from statistical inquiries.
Statistical Significance and P-Values
Statistical significance is a crucial concept in data analysis that indicates whether the result of an analysis is likely to be genuine or a product of random chance. When researchers perform tests, they often calculate a p-value, which expresses the probability of observing the data—assuming the null hypothesis is true. A common threshold to determine significance is 0.05: if the p-value is less than this number, researchers may reject the null hypothesis.
Here are some key aspects to consider:
- Understanding the Null Hypothesis: This is a statement suggesting no effect or no difference, serving as a baseline for comparison.
- Threshold Levels: Often set at 0.05, but depending on the context, more rigorous standards might be applied.
- Misinterpretation Risks: A low p-value does not indicate that an effect is practically significant. Therefore, one must look beyond the p-value itself, considering the context and size of the effect.
"Statistical significance does not guarantee practical relevance. The numbers need a real-world context to create valuable insights."
Confidence Intervals
Confidence intervals provide a range of values that likely encompass the true parameter of interest. For instance, if a study reports a mean income with a 95% confidence interval of $40,000 to $50,000, it implies that there is a 95% chance that the true mean income falls within that span.
Understanding confidence intervals involves several considerations:
- Margin of Error: This defines the range in which the true population parameter is likely to lie. A smaller margin suggests a higher level of precision in the estimate.
- Interpreting Width: Wider intervals might indicate more uncertainty in the estimate, which could stem from sample size or variability in data.
- Reliability Levels: Confidence levels can change the confidence interval. A 99% confidence interval will be wider than a 95% one, reflecting a greater assurance level.
As such, interpreting data is not just about identifying trends but also capturing uncertainty in research conclusions. By appreciating statistical significance and confidence intervals, one can better navigate the intricate waters of data analysis, derive accurate interpretations, and ultimately make more informed decisions.
Limitations and Pitfalls of Data Analysis
Data analysis is a powerful tool, yet it's not without its shadows. Understanding the limitations and pitfalls of data analysis is critically important. Failing to consider these can skew results and lead to misguided decisions. The following sections delve into common errors and conceptual misunderstandings that can arise during the analytical process.
Common Errors in Data Analysis
When we talk about common errors in data analysis, we often mean mistakes that can roll in unnoticed but can pack a punch when it comes to affecting outcomes. These can range from data entry blunders to incorrect assumptions about the dataset. Here are a few prevalent issues:
- Data Entry Mistakes: Sometimes, a simple typographical error, like misspelling a category, can cause significant misinterpretation of results. For instance, if survey data records 'young' and 'yung', it can skew demographic analyses.
- Sampling Bias: This occurs when the sample doesn't represent the broader population. Imagine if a study on consumer behavior only surveyed people in high-income neighborhoods. The insights drawn would be slanted and misleading.
- Overlooking Outliers: Outliers can be both nuisances and goldmines in data. Ignoring an outlier might mean missing a valuable insight, but on the other hand, it can distort statistical summaries. Proper handling and understanding of outliers is crucial.
These errors aren't just footnotes; they can derail an entire project's findings if left unchecked. Attention to detail and a structured validation process can help mitigate these issues.
Overfitting and Underfitting
Moving on to overfitting and underfitting, these two concepts can be like two sides of the same coin in the realm of statistical models. In their nuances, they represent a critical aspect of model selection and data interpretation.
- Overfitting happens when a model learns the details and noise in the training dataset to the extent that it negatively impacts its performance on new data. Picture trying to memorize every word of your favorite book and then find yourself unable to summarize its plot coherently. The model becomes too complex, and while it performs exceptionally well on the training data, it falters in real-world applications.
- Underfitting, on the other hand, is akin to telling a story but leaving out crucial plot points. It happens when a model is too simple to capture the underlying patterns in the data. In attempting to simplify the complexity of the information, meaningful insights can be missed entirely.
Managing these two scenarios requires a balance, often aided by techniques like cross-validation, to ensure that your model generalizes well to unseen data.
"Navigating the landscape of data analysis requires both an understanding of its power and awareness of its limitations. Just as a lighthouse guides ships away from perilous waters, awareness of these pitfalls directs analysts toward reliable insights."
Ultimately, acknowledging limitations in data analysis is paramount. Remember that while data can illuminate many paths, it is crucial to tread carefully. The insights drawn must be interpreted with a discerning eye to help foster sound decision-making.
Ethical Considerations in Data Analysis
When engaging in the realms of statistics and data analysis, the ethical dimensions of these activities cannot be overlooked. Understanding ethical considerations in data analysis involves grappling with responsibilities tied to the collection, interpretation, and reporting of data. Missteps in ethics not only lead to inaccurate insights but can also undermine public trust and violate legal stipulations. As users of data, analysts must tread carefully, considering their actions within the broader landscape of human impact.
Data Privacy Concerns
In today’s digital age, where data flows faster than a river in springtime, privacy concerns are front and center. When conducting data analysis, it's paramount to handle personal information with utmost care. Data privacy isn't merely a good practice; it’s a legal obligation in many jurisdictions like GDPR in the EU or CCPA in California. Analysts must ensure that identifiable information is either anonymized or properly secured to prevent any unauthorized access.
Moreover, transparency is key. Users of data must be informed about what their information is being used for, and consent should always be sought before data collection. These practices not only protect individuals but also enhance the credibility of the data analysis process.
"In the age of data, protection of privacy isn’t just ethical; it’s essential for the very fabric of democratic values."
It’s essential to have structured protocols when it comes to data handling. Analysts might want to consider implementing a clear data governance framework, including regular audits and compliance checks. Failure to do so could lead to significant repercussions, both for individuals and the organizations involved.
Responsible Use of Data
Once data has been collected and processed, the next challenge is its responsible use. Ethical data analysis extends far beyond mere compliance; it hinges on understanding the contextual implications of the data being interpreted. Every dataset tells a story, and it’s the analyst’s responsibility to narrate it truthfully. Misleading representation of data can lead to consequences that may spiral out of control.
When analyzing data, it’s crucial to question the motivations behind its use. Is the data being utilized to benefit society, or does it serve merely personal or corporate interests? Analysts should actively think about how their findings will be framed and used, ensuring that they don’t inadvertently contribute to discriminatory practices or reinforce biases.
Moreover, fostering a culture of ethical responsibility is essential within data-driven organizations. This means encouraging open discussions about ethical dilemmas in data usage amongst team members and integrating ethical guidelines into the data analysis training process.
Consideration of feedback loops can enhance responsibility. Establish channels for stakeholders to express their concerns regarding data use, and don’t shy away from addressing those concerns forthrightly. Doing so not only builds trust with the public but also approximates a robust ethical compass for the future of data analysis.
Overall, balancing the drive for insights with ethical considerations should never be a secondary thought. A commitment to ethical data practices will cement a positive relationship between data analysts and the communities they serve.
Emerging Trends in Statistics and Data Analysis
Emerging trends in statistics and data analysis signify a pivotal shift in how we comprehend and utilize data across various sectors. The modern age is marked by a deluge of information, compelling statisticians and analysts to stay ahead of the curve. Understanding these trends is not just an academic exercise—it's crucial for anyone aiming to decipher the complexities of today’s data-driven landscape. Key elements include technological advancements, real-time analytics, and an accent on collaboration across disciplines, making this topic not only relevant but essential.
Big Data Analytics
Big Data Analytics entails the examination of vast, complex datasets often beyond the capabilities of traditional data processing software. This trend has emerged largely due to the exponential growth of data generated every second. Think of the data produced by social media interactions, transaction processes, or even IoT devices.
Working with big data demands specialized tools and techniques tailored for analyzing such large volumes. For instance, Hadoop and Spark have become popular choices due to their ability to handle big data efficiently. There are various benefits derived from this trend:
- Enhanced Decision-Making: Businesses can derive sharper insights, fueling strategic choices that can make or break their success.
- Predictive Analysis: Companies can foresee trends by analyzing past big data patterns, which leads to proactive strategies rather than reactive ones.
- Customer Insight: With the ability to analyze customer behavior through various touchpoints, firms can tailor their services to meet their clientele’s demands more accurately.
However, the challenge of managing data privacy cannot be overlooked. Big data’s potency often comes with an accompanying responsibility to ensure ethical usage of the information being analyzed.
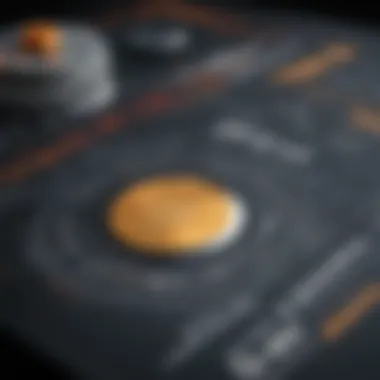
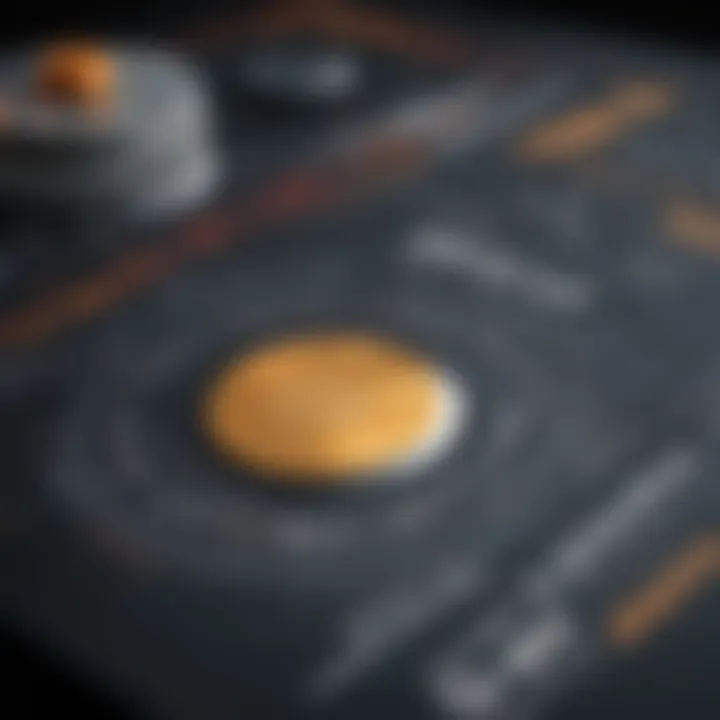
Machine Learning Applications
Machine learning, a subset of artificial intelligence, is revolutionizing statistics and data analysis in remarkable ways. By allowing systems to learn from the data they process, machine learning enables more sophisticated analyses, from anomaly detection to clustering.
These applications are vast, influencing numerous sectors:
- Finance: Algorithms can detect fraudulent transactions by identifying anomalies in spending patterns.
- Healthcare: Predictive models help in diagnosis and treatment recommendations based on patients’ histories.
- Retail: Inventory management systems employ machine learning for stock predictions and automated ordering processes.
The takeaway here is the blend of traditional statistical methods with cutting-edge technology. By allowing machines to take the reins on routine analyses, data scientists can devote their energies to more complex problems, which typically require human intuition and expertise.
The Role of Software in Data Analysis
In today’s data-driven landscape, the role of software in data analysis cannot be overstated. The sheer volume of data generated daily is staggering, and analyzing this data efficiently often requires sophisticated tools. Software helps in streamlining workflows, ensuring accuracy, and enhancing the overall analytical process. This section examines the importance of software, emphasizing specific elements that elevate data analysis from mere numbers to insightful narratives.
The utilization of software in data analysis signifies not just a convenience but a necessity. From simple statistical tasks to complex predictive modeling, the right tools can dramatically influence outcomes. They empower users to handle vast datasets, automate repetitive tasks, and apply a variety of statistical methods with relative ease. As anyone who’s ever dabbled in data analysis knows, the ability to visualize results and interpret them accurately is often half the battle won. Here, we also explore considerations such as cost, accessibility, and user-friendliness of these tools.
"Software doesn't just ease the process; it transforms the entire landscape of data analysis into a manageable and efficient endeavor."
Statistical Software Overview
Statistical software serves as the bedrock of modern data analysis practices. It includes a variety of programs designed to facilitate statistical computations, data manipulation, and visualization. Some of the most well-known statistical packages include SAS, SPSS, and R among others. Each of these software packages comes with its own set of features tailored to meet the needs of analysts.
- SAS (Statistical Analysis System): Renowned for its robust statistical capabilities and data management features, SAS is often favored by large organizations needing comprehensive analytics solutions.
- SPSS (Statistical Package for the Social Sciences): Commonly used in social science research, SPSS is known for its ease of use and strong support for a wide range of statistical techniques.
- R: As an open-source programming language, R is a go-to for statisticians and data scientists due to its versatility and the extensive library of packages available for various types of analysis.
Choosing the right software depends on a variety of factors including the specific needs of the project, the analyst’s familiarity with the tool, and budget constraints. The growing emphasis on analytical skills has also led to increased demand for educational resources that help in navigating these tools effectively.
Open Source vs. Proprietary Tools
When discussing the role of software in data analysis, a critical consideration often arises — open source vs. proprietary tools. Both types of software have their own advantages and limitations, catering to different user needs and preferences.
- Open Source Tools
- Proprietary Tools
- Cost-effective: Open-source tools are freely available, significantly reducing software costs, especially for startups and independent analysts.
- Community Support: With large communities backing popular open-source projects like R or Python, users can benefit from shared knowledge and continuous updates.
- Customization: Open-source software allows users to modify the source code to tailor functionalities to their specific needs.
- User-Friendly Interfaces: Proprietary software often features intuitive interfaces, making them easier to navigate — a big plus for beginners who may feel overwhelmed.
- Comprehensive Support: Companies providing proprietary tools usually offer customer service and support, which can be crucial for businesses needing timely assistance.
- Advanced Features: Proprietary tools may offer advanced analytics capabilities that are polished and ready for immediate application, reducing the time needed for implementation.
Ultimately, the choice between open-source and proprietary software hinges on the project's scope, the technical skills of the user, and the specific requirements for analysis. Recognizing the strengths and weaknesses of each option allows analysts to make informed decisions that best suit their objectives.
Case Studies in Data Analysis
In today’s fast-paced world, statistical data analysis is more than just numbers and computations. It’s about storytelling through data. Case studies in data analysis stand as pivotal elements in demonstrating how real-world applications yield insights or transform businesses. This section discusses the essence of case studies, emphasizing their benefits and considerations.
Real-world Applications
Case studies serve as a bridge between theory and practice. They illustrate how various industries effectively utilize data analysis to drive decision-making. Take, for instance, the retail sector. A large clothing retailer might analyze customer purchasing patterns to optimize inventory. By examining past sales data, they could identify trends in color preferences or sizes. Consequently, they adjust their stock accordingly, which minimizes excess inventory and maximizes sales.
In healthcare, data analytics aids in patient outcome improvements. Hospitals use data to analyze readmission rates. By investigating these cases, management can pinpoint the root causes, leading to enhanced patient care protocols. This exemplifies the practical implications of data analysis, providing substantial benefits not only to institutions but to society as a whole.
Here are other notable applications:
- Marketing Strategies: Companies analyze data from social media campaigns to refine their strategies, improving audience engagement.
- Financial Forecasting: Financial firms use historical data to predict market trends and mitigate risks associated with investments.
- Manufacturing Efficiency: Manufacturers employ data analytics to streamline operations, reduce waste, and improve production processes.
These examples underscore the vast potential of data analysis across various sectors, showcasing its importance in guiding strategic choices.
Lessons Learned from Case Studies
The beauty of case studies is they offer valuable lessons that can be applied broadly. One of the key takeaways is the importance of data quality. Poor data collection methods lead to skewed results and, ultimately, misguided decisions. For instance, a case involving a restaurant chain showed how inconsistent customer feedback data led to misjudgments about menu changes. After revising their data collection methods, they observed improvements in customer satisfaction.
Another crucial lesson revolves around the concept of adaptability. Businesses must learn to pivot based on the insights they gather. For example, an e-commerce platform recognized a shift in consumer behavior during the pandemic. By analyzing web traffic data, they adapted their offerings to cater to the emerging needs of their customers. This adaptability not only preserved their sales but also positioned them favorably in a competitive market.
Moreover, case studies often reveal the necessity of cross-functional collaboration. In one notable instance, a telecommunications company combined efforts between its IT and marketing teams to analyze customer usage data effectively. This partnership led to targeted offerings that increased customer retention rates significantly.
"Data analysis is the compass that helps businesses navigate the uncertain waters of decision-making."
In essence, the lessons derived from case studies in data analysis highlight a critical aspect of modern business: the art of learning from both successes and setbacks. Through these explorations, organizations can refine strategies and uncover opportunities for growth.
Future of Statistics Data Analysis
The landscape of statistics and data analysis is on the verge of significant evolution, driven by both advancements in technology and the growing demands for more rigorous data interpretation. This future is not just about employing better tools, but also about how we conceptualize analysis itself. As data becomes more abundant and complex, the methodologies we utilize must adapt to capture deeper insights and ensure the responsible use of information.
In this ever-changing field, there are a few elements that stand out:
- Innovative methodologies: As new techniques are developed, traditional methods may integrate to enhance accuracy and depth of insights.
- Interdisciplinary collaboration: Different fields are beginning to merge their expertise, creating robust data analysis methods that benefit multiple sectors.
- Ethical responsibility: The importance of maintaining ethical standards in data collection and analysis is central to cultivating trust in statistical insights.
"The future of data analysis is not just in the data, but in the interpretation and ethical considerations behind it."
Predictions for the Next Decade
Looking down the road, several predictions emerge about what we can expect in statistics and data analysis. First off, the rise of automated analytics will simplify the interpretation process. With automation, non-experts will find it easier to generate insights, thereby democratizing data access.
- Increased use of AI and Machine Learning: As these technologies evolve, they will likely handle larger datasets with greater flexibility and speed. This could allow for more complex analyses that were previously unattainable.
- Real-time data analysis: The urge for up-to-minute insights is ever-growing. The capacity to analyze data as it happens will create significant shifts in how businesses respond to changes.
- Enhanced visualization technologies: The future will see more immersive data visualization techniques, possibly incorporating VR (Virtual Reality) or AR (Augmented Reality) to help users grasp complex data landscapes with ease.
Adapting to Technological Changes
Given the rapid pace of technological progress, adapting is essential. Organizations will need to embrace ongoing learning and stay updated with emerging trends in data analysis tools.
- Digital literacy: As analytics become increasingly integrated into business operations, understanding the fundamental concepts of data analysis will be crucial for more professionals, not just analysts.
- Integration of cloud-based solutions: The shift towards cloud technology allows for enhanced collaboration among teams, facilitating more versatile and agile data analysis practices.
- Continual evaluation: Companies should regularly assess whether their methods and tools are aligned with technological advancements, ensuring they aren't left riding the coattails of previous eras.
The future of statistics data analysis lies in a harmonious blend of technology, skill enhancement, and ethical approaches. Embracing this multifaceted future will set the stage for insightful and responsible data interpretations.
Finale
Wrapping up, the conclusion serves as the cornerstone of this exploration into statistics data analysis. It's not just a neat bow on the package; it emphasizes the importance of understanding the facets of data analysis we’ve navigated. The key insights gleaned throughout our journey reveal how critical data interpretation is in making informed decisions across various fields ranging from finance to healthcare.
Summarizing Key Insights
To distill the numerous discussions we’ve had, let’s highlight several crucial points:
- Diverse Statistical Methods: Both descriptive and inferential statistics serve distinct purposes but are equally vital for accurate data interpretation.
- Impact of Visualization: Effective data visualization can significantly enhance the ability to discern patterns and make data-driven decisions.
- Potential Pitfalls: Being aware of common errors in data analysis, such as biases and overfitting, can help maintain analytical integrity.
- Ethical Implications: The need for responsible data handling and respect for privacy stands out as a mandate in today’s data-centric world.
In summation, every statistic tells a story, and every analysis paves the road for deeper understanding and better decision-making. 🔍
Encouragement for Further Study
As we conclude, I urge readers—whether you're just kicking the tires or well-versed in the field—to further delve into the intricacies of statistics data analysis. The domain is ever-evolving and infinitely rich.
- Stay Updated: Subscribe to journals or websites that focus on advancements in data analysis, like those found on Wikipedia and Britannica.
- Engage in Community Discussions: Platforms like Reddit can be treasure troves of knowledge, offering insights from practitioners who are knee-deep in the data trenches.
- Practical Applications: Seek opportunities to apply learned techniques in real-world scenarios—internships, projects, or even personal experiments with publicly available datasets can serve wonders for honing your skills.
A commitment to ongoing education will not only enhance your analytical abilities but can also unravel new perspectives on the staggering amount of data that informs our world today.